One of the main goals of artificial intelligence (AI) is to replicate the way the human brain thinks. For decades, computer scientists have worked on improving AI systems, and in recent times, real-world applications are making an impact on businesses. Cybersecurity is one such field.
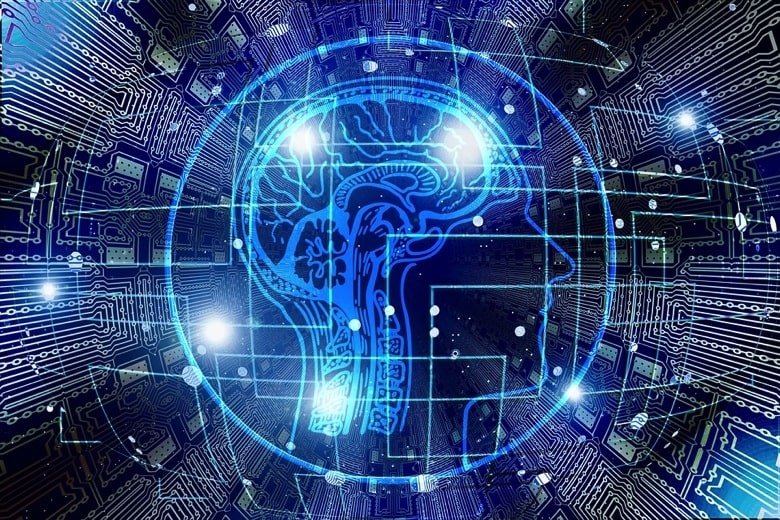
Interestingly, though, humans are considered to be a weak link in cybersecurity, because we are vulnerable to social engineering, insider malicious activity, and even simple bad security habits. Thus, AI is providing a new perspective in addressing these security risks and threats. With AI, systems learn and work autonomously. Security solutions are not only able to address threats automatically, but they also improve and learn as they are exposed to new threats and attacks.
What is user and entity behavior analytics?
One of the ways by which AI is utilized to keep track of threats is through user and entity behavior analytics (UEBA), which learns how people normally conduct their business within a secured system. This relatively new security solution employs AI to identify anomalous behaviors and decide if these will result in security implications. If there is risky behavior, then security teams are alerted for action.
In contrast, rules-based threat detection analyzes movements and matches these with predefined relational models. It is only effective for known threats, however. It will be rendered weak in cases where the attacks are new or unknown. Rules or correlation will not be effective against insider threats, too, as these are often interpreted as normal user activity by traditional security systems.
By determining suspicious activities without relying solely on information about previous activities, predefined patterns, and sets of rules, artificial intelligence provides a notable improvement in identifying security issues.
How does it work?
The way UEBA operates can be summarized by the following steps: data collection, establishing normal user behavior patterns, analysis, and continuous monitoring of users and entities to compare them to normal behavior.
Just like any other system that uses artificial intelligence or machine learning, it’s important to collect data. Typically, UEBA solutions are fed with data compiled in a general data repository such as a data warehouse, data lake, or security information and event management (SIEM) system. The data collected include event and system logs, network flows and packets, business context, HR and user context, and external threat intel.
Once all the required data are collected, the baselining stage follows. Baselining involves establishing what constitutes normal behavior. It is an essential function of UEBA, which sets the standards of normalcy that will be used to detect anomalies. These standards or models of normal behavior patterns are determined using advanced analytical methods. The system goes through a multitude of use instances to obtain enough information that can conclusively determine what normal user and entity behaviors are. The UEBA system then calculates risk scores and sets deviations that can be considered acceptable.
After the baselining stage, the system proceeds to monitor and analyze activities. This is where AI, particularly machine learning, is employed along with statistical models, rules, and threat signatures. Using the risk scores and deviation thresholds set during baselining, the system evaluates user behaviors to determine if they are normal or anomalous.
Machine learning can be both unsupervised and supervised. The system learns as it encounters new information and absorbs rules, statistical models, and threat signatures. Over time, the system learns and improves its ability to detect threats as it interfaces with more of them. Security experts who handle the system can also directly introduce rules and models. The system can also integrate deep learning, ensemble networks, and generative adversarial networks to enhance its capabilities.
The analytical stage looks at various use cases to identify possible malicious insiders, compromised users, as well as advanced persistent threats and zero-day vulnerabilities. To emphasize, UEBA solutions are designed to be applicable to multiple use cases. They do not focus on specialized aspects of cybersecurity like fraud detection and monitoring of trusted hosts. With the help of AI, the system can perform a more comprehensive analysis and cover all emerging and evolving threats that specifically target people.
Establishing context
Coupled with big data analytics, artificial intelligence enables accurate framing of data in relation to events, users, and devices. Context will make data useful and ensure the correct application of rules and standards in determining whether a behavior is potentially harmful or not. It aids in identifying threats and in meaningful automation of certain processes in threat detection.
Without context, false positives will distort automated threats detection. This can adversely impact the viability of using automation in threat analysis and prevention. The lack of context also stifles machine learning. AI-powered security systems cannot set clear distinctions of normal and irregular behavior without context.
Identifying human weaknesses, but not replacing people
Humans are often regarded as the weakest link in cybersecurity. People or users are the ones who create the opening for attacks, whether intentionally or unwittingly. Cybercriminals are often successful in using phishing and other social engineering tactics to trick people into doing things that result in vulnerabilities or facilitate penetration. The number of successful social engineering penetrations has been growing. According to a CyberEdge report, the success rate of social engineering attacks in 2017 was at 79%, an increase from 76% in 2016, 71% in 2015, and 62% in 2014. It’s only logical to do something to arrest this trend.
Weaknesses in security systems are inevitable as long as people are involved, but they can be mitigated. AI, however, is not used to replace or eliminate human involvement in a security system. Rather, AI serves as an objective assessor of human behavior, in order to inform security professionals if the actions are risky. AI simulates human scrutiny in determining whether a behavior is normal or anomalous. The process is automated, resulting in greater efficiency and improved consistency.
In conclusion
AI may replace people in automating repetitive processes in threat detection. However, its goal is not to take people out of the picture. The use of artificial intelligence in cybersecurity is an excellent example of how technology augments people’s capabilities, not replace them. AI enhances security solutions by using reasoning and relationship algorithms to identify and predict risks. It imitates the human ability to establish baselines of normal behavior, see context, and decide if a behavior or pattern of activities is normal or a manifestation of an attack. AI boosts security solutions as it addresses vulnerabilities associated with human actions and decision making.
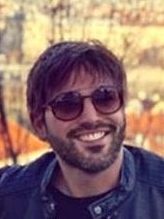
Cyber Security Researcher. Information security specialist, currently working as risk infrastructure specialist & investigator. He is a cyber-security researcher with over 25 years of experience. He has served with the Intelligence Agency as a Senior Intelligence Officer. He has also worked with Google and Citrix in development of cyber security solutions. He has aided the government and many federal agencies in thwarting many cyber crimes. He has been writing for us in his free time since last 5 years.